Understanding JRockit VM:Adaptive Memory Management
This post is talking about automatic and adaptive memory management in the Java runtime. It mainly includes the following content: concepts of memory management, how a garbage collector works, memory management in the JRockit VM.
Concepts of Automatic Memory Management
Automatic memory management is defined as any garbage collection technique that automatically gets rid of stale references, making a free operator unnecessary.
There are many implementation of automatic memory management techniques, such as reference counting, heap management strategies, tracing techniques (traversing live object graphs).
Adaptive memory management
JVM behavior on runtime feedback is a good idea. JRockit was the first JVM to recognize that adaptive optimization based on runtime feedback could be applied to all subsystems in the runtime and not just to code generation. One of these subsystems is memory management.
Adaptive memory management is based heavily on runtime feedback. It is a special case of automatic memory management.
Adaptive memory management must correctly utilize runtime feedback for optimal performance. This means changing GC strategies, automatic heap resizing, getting rid of memory fragmentation at the right intervals, or mainly recognizing when it is most appropriate to “stop the world”. Stopping the world means halting the executing Java program, which is a necessary evil for parts of a garbage collection cycle.
Advantages of automatic memory management
- It contributes to the speed of the software development cycle. Because using automatic memory management, memory allocation bugs can’t occur and buffer overruns can’t occur.
- An adaptive memory manager may pick the appropriate garbage collection strategy for an application based on its current behavior, appropriately changing the number of garbage collecting threads or fine tuning other aspects of garbage collection strategies whenever needed.
Disadvantages of automatic memory management
- It can slow down execution for certain applications to such an extent that it becomes impractical.
- There may still be memory leaks in a garbage collected environment.
Fundamental Heap Management
Before knowing actual algorithms for garbage collection, we need to know the allocation and deallocation of objects. We also need to know which specific objects on the heap to garbage collect, and how they get there and how they are removed.
Allocating and releasing objects
Allocation on a per-object, in the common case, never takes place directly on the heap. Rather, it is performed in the thread local buffers or similar constructs that promoted to the heap from time to time. However, in the end, allocation is still about finding appropriate space on the heap for the newly allocated objects or collections of objects.
In order to put allocated objects on the heap, the memory management system must keep track of which section of the heap are free. Free heap space is usually managed by maintaining a free list – a linked list of the free memory chunks on the heap, prioritized in some order that makes sense.
A best fit or first fit can be performed on the free list in order to find a heap address where enough free space is available for the object. There are many different allocation algorithms for this, with different advantages.
Fragmentation and compaction
It is not enough to just keep track of free space in a useful manner. Fragmentation is also an issue for the memory manager. When dead objects are garbage collected all over the heap, we end up with a lot of holes from where objects have been removed.
Fragmentation is a serious scalability issue for garbage collection, as we can have a very large amount of free space on the heap that, even though it is free, is actually unusable.
If the memory manager can’t find enough contiguous free space for the new object, an OutOfMemoryError
will be thrown, even though there are much free space on the heap.
Thus, a memory management system needs some logic for moving objects around on the heap, in order to create larger contiguous free regions. This process is called compaction, and involves a separate GC stage where the heap is defragmented by moving live objects so that they are next to one another on the heap.
Compaction is difficult to do without stopping the world. By looking at the object reference graph and by gambling that objects referencing each other are likely to be accessed in sequence, the compaction algorithm may move these objects so that they are next to one another on the heap. This is beneficial for the cache, the object lifetimes are similar so that larger free heap holes are crated upon reclamation.
Garbage collection algorithms
All techniques for automatic memory management boil down to keeping track of which objects are being used by the running program, in other words, which objects are referenced by other objects that are also in use. Objects that are no longer in use may be garbage collected. Objects in use also means live objects.
There are two category common garbage collection techniques: reference counting and tracing garbage collection.
Reference counting
Reference counting is a garbage collection algorithm where the runtime keeps track of how many live objects point to a particular object at a given time.
When the reference count for an object decreases to zero, the object has no referrers left, the object is available for garbage collection.
Advantages of reference counting algorithm
- It is obvious simplicity, is that any unreferenced object may be reclaimed immediately when its reference count drops to zero.
Disadvantages of reference counting algorithm
- The obvious flaw that cyclic constructs can never be garbage collected. Consequently cause a memory leak.
- Keeping the reference counts up to date can be expensive, especially in a parallel environment where synchronization is required.
There are no commercial Java implementations today where reference counting is a main garbage collection technique in the JVM, but it might well be used by subsystems and for simple protocols in the application layer.
Tracing techniques
A tracing garbage collection start by marking all objects currently seen by the running program as live. The recursively mark all objects reachable from those objects live as well. There are many variations of tracing techniques, for example, “mark and sweep” and “stop and copy”.
There are some basic concepts of tracing techniques, for example, root set that means the initial input set for this kind of search algorithm, that is the set of live objects from which the trace will start. The root set contains all objects that are available without having to trace any references.
Mark and sweep
The mark and sweep algorithm is the basis of all the garbage collectors in all commercial JVMs today. Mark and sweep can be done with or without copying or moving objects. However, the real challenge is turning it into an efficient and highly scalable algorithm for memory management. The following pseudocode describes a naive mark and sweep algorithm:
Mark: |
The following figures show the process of mark and sweep:
- Before mark
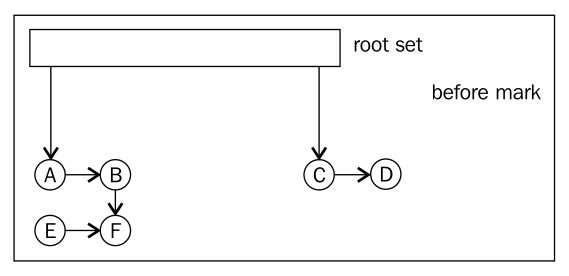
- After mark.
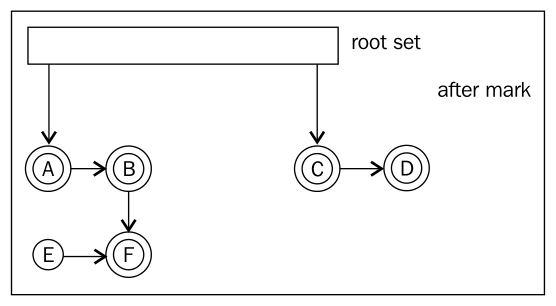
- After sweep
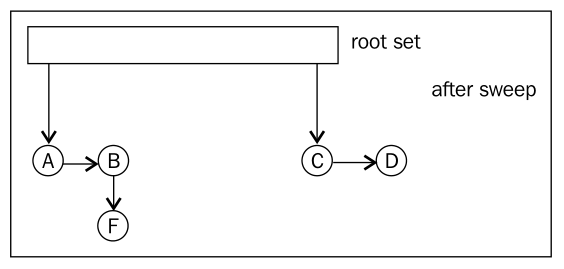
In naive mark and sweep implementations, a mark bit is typically associated with each reachable object. The mark bit keeps track of if the object has been marked or not.
A variant of mark and sweep that parallelizes better is tri-coloring mark and sweep. Basically, instead of using just one binary mark bit per object, a color, or ternary value is used. There are three color: white, grey, and black.
- White objects are considered dead and should be garbage collected.
- Black objects are guaranteed to have no references to white objects.
- Grey objects are live, but with the status of their children unknown.
Initially, there are no black object – the marking algorithm needs to find them, and the root set is colored grey to make the algorithm explore the entire reachable object graph. All other objects start out as white.
The tri-color algorithm is fairly simple:
Mark: |
The main idea here is that as long as the invariant that no block nodes ever point to white nodes is maintained, the garbage collector can continue marking even while changes to the object graph take place.
Stop and Copy
Stop and copy can be seen as a special case of tracing GC, and is intelligent in its way, but is impractical for large heap sizes in real applications.
Stop and copy garbage collection partitioning the heap into two region of equal size. Only one region is in use at a time. Stop and copy garbage collection goes through all live objects in one of the heap regions, starting at the root set, following the root set pointers to other objects and so on. The marked live objects are moved to the other heap region. After garbage collection, the heap regions are switched so that other half of the heap becomes the active region before the next collection cycle.
Advantages of stop and copy algorithm:
- fragmentation can’t become an issue.
Disadvantages of stop and copy algorithm:
- All live objects must be copied each time a garbage collection is performed, introducing a serious overhead in GC time as a function of the amount of live data.
- Only using half of heap at a time is a waste of memory.
The following figure shows the process of stop and copy:
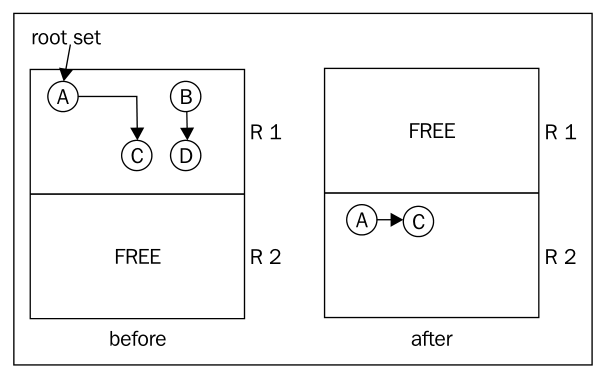
Generational garbage collection
In object-oriented languages, most objects are temporary or short-lived. However, performance improvement for handling short-lived objects on the heap can be had if the heap is split into two or more parts called generations.
In generational garbage collection, new objects are allocated in “young” generations of the heap, that typically are orders of magnitude smaller than the “old” generation, the main part of the heap. Garbage collection is then split into young and old collections, a young collection merely sweeping the young spaces of the heap, removing dead objects and promoting surviving objects by moving them to an older generation.
Collecting a smaller young space is orders of magnitude faster than collecting the larger old space. Even though young collections need to happen far more frequently, this is more efficient because many objects die young and never need to be promoted. ideally, total throughput is increased and some potential fragmentation is removed.
JRockit refer to the young generations as nurseries.
Muti generation nurseries
While generational GCs typically default to using just one nursery, sometimes it can be a good idea to keep several small nursery partitions in the heap and gradually age young objects, moving them from the “younger” nurseries to the “older” ones before finally promoting them to the “old” part of heap. This stands in contrast with the normal case that usually involves just one nursery and one old space.
Multi generation nurseries may be more useful in situations where heavy object allocation takes place.
If young generation objects live just a bit longer, typically if they survive a first nursery collection, the standard behavior of a single generation nursery collector, would cause these objects to be promoted to the old space. There, they will contribute more to fragmentation when they are garbage collected. So it might make sense to have several young generations on the heap, with different age spans for young objects in different nurseries, to try to keep the heap holes away from the old space where they do the most damage.
Of course the benefits of a multi-generational nursery must be balanced against the overhead of copying objects multiple times.
Write barriers
In generational GC, objects may reference other objects located in different generations of the heap. For example, objects in the old space may point to objects in the young spaces and vice versa. If we had to handle updates to all references from the old space to the young space on GC by traversing the entire old space, no performance would be gained from the generational approach. As the whole point of generational garbage collection is only to have to go over a small heap segment, further assistance from the code generator is required.
In generational GC, most JVMs use a mechanism called write barriers to keep track of which parts of the heap need to be traversed. Every time an object A starts to reference another object B, by means of B being placed in one of A’s fields or arrays, write barriers are needed.
The traditional approach to implementing write barriers is to divide the heap into a number of small consecutive sections (typically about 512 bytes each) that are called cards. The address space of the heap is thus mapped to a more coarse grained card table. whenever the Java program writes to a field in an object, the card on the heap where the object resides is “dirtied” by having the write barrier code set a dirty bit.
Using the write barriers, the traversal time problem for references from the old generation to the nursery is shortened. When doing a nursery collection, the GC only has to check the portions of the old space represented by dirty cards.
Throughput versus low latency
Garbage collection requires stopping the world, halting all program execution, at some stage. Performing GC and executing Java code concurrently requires a lot more bookkeeping and thus, the total time spent in GC will be longer. If we only care about throughput, stopping the world isn’t an issue–just halt everything and use all CUPs to garbage collect. However, to most applications, latency is the main problem, and latency is caused by not spending every available cycle executing Java code.
Thus, the tradeoff in memory management is between maximizing throughput and maintaining low latencies. But, we can’t expect to have both.
Garbage collection in JRockit
The backbone of the GC algorithm used in JRockit is based on the tri-coloring mark and sweep algorithm. For nursery collections, heavily modified variants of stop and copy are used.
Garbage collection in JRockit can work with or without generations, depending on what we are optimizing for.
Summary
- First, we need to know what is automatic memory management, its advantages and disadvantages.
- Fundamentals of heap management are: how to allocating and releasing objects on the heap, how to compact fragmentations of heap memory.
- There are two common garbage collection algorithms: reference counting and tracing garbage collection. The reference counting is simple, but have some serious drawbacks. There are many variations of tracing techniques: “mark and sweep” and “stop and copy”. The “stop and copy” technique has some drawbacks, and it only uses in some special scenarios. Therefore, the mark and sweep is the most popular garbage collection algorithm use in JVMs.
- The real-world garbage collector implementation is called generational garbage collection that is based on the “mark and sweep” algorithm.
References
[1] Oracle JRockit: The Definitive Guide by Marcus Hirt, Marcus Lagergren